Unlocking Potential with a Machine Learning Labeling Tool
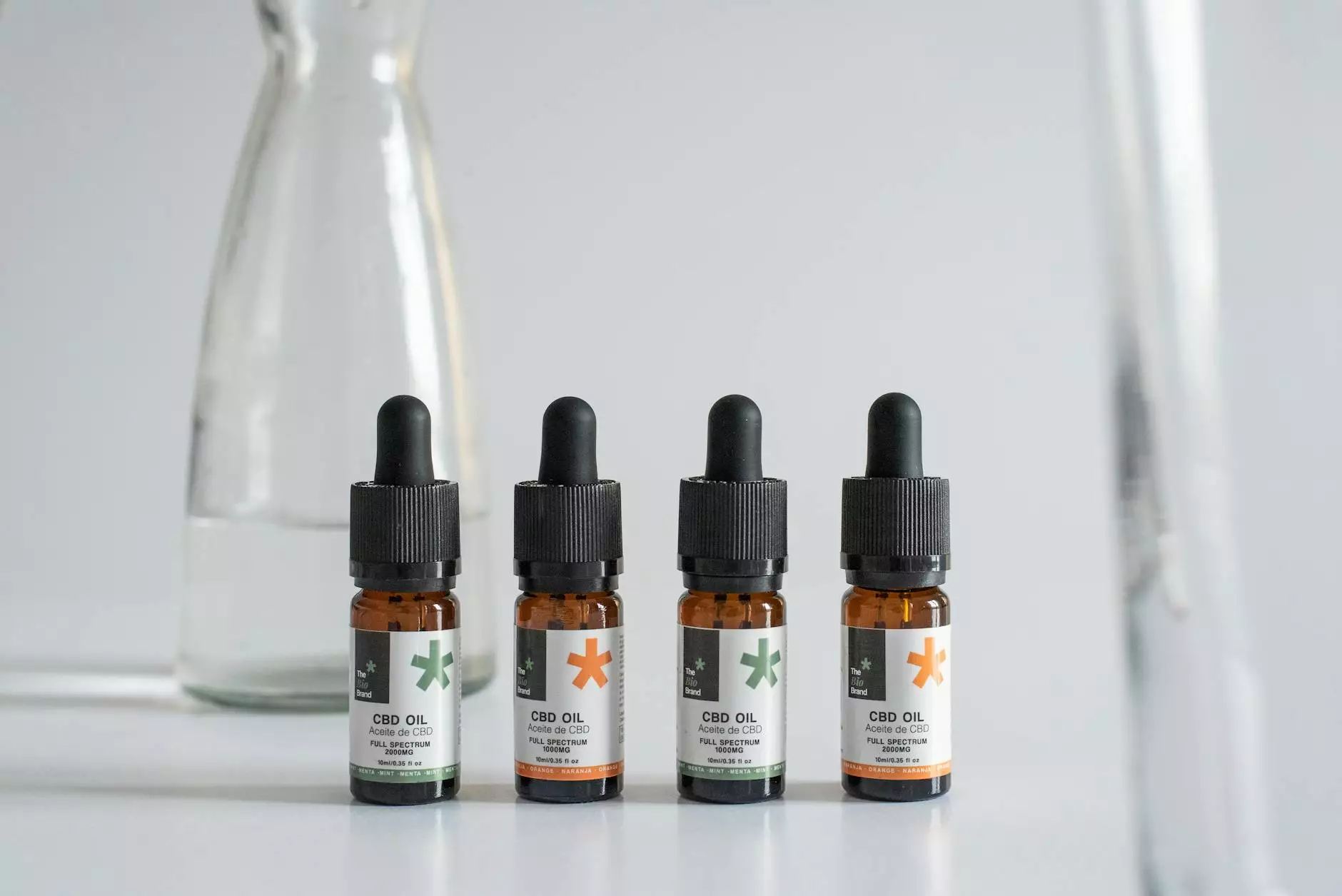
In the age of artificial intelligence and big data, the importance of high-quality data annotation cannot be overstated. Data serves as the backbone for machine learning algorithms, and its effectiveness is determined significantly by how well it is labeled and categorized. This is where a machine learning labeling tool comes into play, streamlining the annotation process while ensuring maximum accuracy and efficiency.
Understanding Data Annotation
Data annotation is the process of labeling data to train machine learning models effectively. It involves providing metadata that can be used to identify patterns, features, or objects within the dataset. This can include:
- Image Annotation: Labeling images for object detection, image segmentation, or facial recognition.
- Text Annotation: Tagging parts of speech, entities, or sentiment in text data.
- Audio Annotation: Marking specific sounds or transcribing spoken words.
The Role of a Machine Learning Labeling Tool
A proper machine learning labeling tool enhances the data annotation process by providing advanced functionalities that allow users to:
- Improve annotation speed through efficient user interfaces.
- Ensure consistency by applying pre-defined labeling rules.
- Reduce human error with automated suggestions and checks.
- Scale annotation projects quickly to meet growing data needs.
Key Features of a Machine Learning Labeling Tool
Choosing the right machine learning labeling tool for your business depends on its features. Here are some essential components to consider:
User-Friendly Interface
An intuitive user interface is crucial for ensuring that both technical and non-technical users can efficiently navigate the tool. A good labeling tool will provide a clean layout, easy access to features, and simple navigation to enhance the productivity of the annotators.
Customizable Workflows
Every project is unique, and a one-size-fits-all approach can lead to inefficiencies. Machine learning labeling tools should offer customizable workflows that allow users to define specific annotation processes tailored to their project needs.
Support for Multiple Data Types
A versatile machine learning labeling tool should support different types of data, including images, text, videos, and audio. This ensures that users can work with diverse datasets within a single platform, simplifying the management of data annotation projects.
Quality Assurance Mechanisms
To maintain a high level of data accuracy, effective quality assurance mechanisms are essential. These may include:
- Automated Quality Checks: Tools that automatically verify the consistency and correctness of annotations.
- Review Systems: Allowing multiple annotators to review and approve each other's work ensures high-quality outcomes.
- Version Control: Tracking changes in annotations to avoid confusion and maintain data integrity.
The Benefits of Using a Machine Learning Labeling Tool
Implementing a machine learning labeling tool can yield several benefits for organizations:
Enhanced Efficiency
The speed of data annotation significantly increases with the use of these tools. Processes that could take weeks can be completed in days, allowing organizations to bring their machine learning projects to fruition faster.
Cost Reduction
By streamlining the annotation process, businesses can reduce labor costs associated with hiring extensive teams of data annotators. Automation and ease of use lead to lower overhead costs while maintaining high-quality output.
Scalability
As data needs grow, businesses can scale their annotation projects without sacrificing quality. A reliable machine learning labeling tool can handle larger datasets, making it easier to adapt to changing circumstances.
Improved Collaboration
Many modern tools facilitate collaboration among teams, allowing members to work together on projects seamlessly. This improved communication enhances overall teamwork and leads to better outcomes.
Choosing the Right Machine Learning Labeling Tool
When selecting a machine learning labeling tool, consider the following factors:
Integration Capabilities
Ensure that the tool can integrate with other platforms and software used in your organization. This compatibility can save time and effort during the data processing stages.
Support and Community
Look for tools that offer robust customer support and active user communities. A strong support system ensures that you can resolve any issues quickly and find help when needed.
Pricing Structure
Evaluate the pricing model and ensure it aligns with your budget. Many tools offer various pricing plans based on features, so choose one that balances cost with your specific requirements.
Real-World Applications of Machine Learning Labeling Tools
Organizations across various industries are leveraging machine learning labeling tools to enhance their operations:
Healthcare
In the healthcare sector, data annotation can be used to train algorithms for image analysis, helping in the early detection of diseases by analyzing X-rays and MRI scans.
Autonomous Vehicles
Data annotation is critical for training machine learning models that drive autonomous vehicles. Precise labeling of objects in images, such as pedestrians, road signs, and obstacles, is fundamental for safe navigation.
Finance
In finance, machine learning labeling tools help annotate transaction data, improve fraud detection models, and develop algorithms for stock market predictions.
The Future of Data Annotation with Machine Learning Tools
The field of data annotation is rapidly evolving with advancements in technology. Machine learning labeling tools are expected to become more sophisticated, incorporating AI-driven features that further automate the labeling process and improve accuracy.
AI-Assisted Annotation
Future tools may utilize AI to suggest annotations automatically, significantly reducing the time required for manual input. This could lead to enhanced productivity and allow human annotators to focus on more complex tasks.
Real-Time Collaboration
As remote work becomes more common, tools that allow for real-time collaboration among distributed teams will become essential. Enhanced features that enable team communication and instant feedback will shape the future of data annotation.
Conclusion
In conclusion, adopting a machine learning labeling tool is not merely a smart business decision; it is a necessary step for organizations aiming to remain competitive in an increasingly data-driven world. By improving efficiency, reducing costs, and enhancing data quality, these tools pave the way for successful machine learning initiatives. As the technology advances, the future holds even greater potential for innovation and application across various industries.
Companies like Keylabs.ai offer cutting-edge solutions in the realms of data annotation tools and platforms. Investing in the right tool will not only streamline your processes but will also empower your organization to harness the full potential of artificial intelligence. Start your journey today with a robust machine learning labeling tool and watch your capabilities soar.